2013
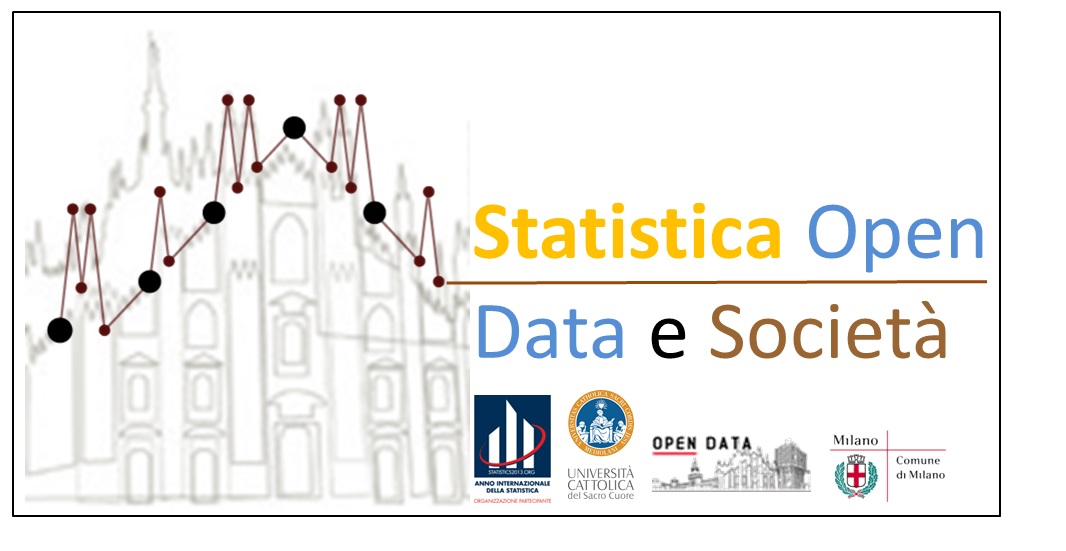
AL VIA IL CONCORSO A PREMI PROMOSSO DA COMUNE DI MILANO
E UNIVERSITA' CATTOLICA SULLA VALORIZZAZIONE DEGLI OPEN DATA
C'è un tesoro nascosto nei dati. La statistica può aiutarti a trovarlo.
Comune di Milano e Università Cattolica del Sacro Cuore, in occasione dell'anno internazionale della Statistica 2013, lanciano il concorso a premi "Statistica, Open Data e Società" per il miglior uso degli Open Data ai fini di migliorare la conoscenza della realtà cittadina ed accrescere il benessere collettivo.
Gli Open Data sono sempre più riconosciuti come un'immensa risorsa a disposizione dei cittadini, con un potenziale ancora tutto da sfruttare. Un tesoro, appunto, da scoprire e da valorizzare con gli strumenti adatti che la statistica - disciplina che produce conoscenza attraverso i dati - può mettere a disposizione.
Ad oggi (dati aggiornati a giugno 2013) sono presenti in Italia oltre 6700 dataset rilasciati in formato aperto da oltre 70 amministrazioni (dati.gov.it). Un valore triplicato nell'ultimo anno e mezzo a testimonianza della forte crescita e del vasto interesse pubblico nei confronti di questi dati. Il portale Open Data del Comune di Milano (dati.comune.milano.it/) è nato ad ottobre 2012 ed ha al momento già superato i 150 dataset disponibili e scaricabili su vari temi (economia, demografia, istruzione, trasporto pubblico, tempo libero, ecc.).
Il concorso prevede 3 premi di 1000 euro ciascuno. Verranno presi in considerazione articoli, infografiche, rapporti di ricerca, video, o qualsiasi altro prodotto (tecnologico, di testo o grafico) che almeno in una sua parte contenga una elaborazione di dati scaricati dal portale Open Data del Comune di Milano.
La valutazione terrà conto dei seguenti aspetti: creatività ed originalità; contenuto informativo del prodotto anche in termini di conoscenza della realtà cittadina; adeguatezza e pertinenza del trattamento dei dati.
Nel portale dedicato www.unicatt.it/concorsoOpenData2013 si può trovare il regolamento e ogni altra informazione utile per la partecipazione al concorso. La scadenza per l'invio delle domande con allegato il prodotto per la valutazione è fissata all'11 novembre 2013.
La premiazione avverrà nel corso di un evento pubblico previsto nel mese di dicembre 2013 nel quale si dibatteranno, con esperti e decisori pubblici, i temi legati all'importanza degli Open Data e della statistica per la conoscenza e il miglioramento della realtà cittadina.
Sostengono l'iniziativa Synergia s.r.l. e Nunatac s.r.l. (finanziatori dei premi) e Linkiesta (media partner).
Comunicati stampa:
Prof.ssa Chiara TOMMASI
Venerdì 13 Dicembre 2013
An introduction to optimal design of experiments
Experiments are commonly conducted in many scientific contexts. In this setting the experimenter can freely choose the levels of some experimental conditions X; at each value of X an experiment is run and a response variable Y is observed.
Let the probability distribution of Y (i.e. the statistical model) depend on the experimental conditions and let it be completely known except for some parameters.
The goal of the Theory of Optimum Design is to fix the levels of the experimental conditions and the proportion of observations to be taken at each level, in order to estimate as precisely as possible the unknown parameters of the model. These optimal levels and proportions of observations constitute an optimal design. Optimal designs are computed minimizing some convex functions (called optimality criteria) of the inverse of the Fisher information matrix. Therefore, an optimal design minimizes (in some sense) the asymptotic covariance matrix of the maximum likelihood estimator. The most popular optimality criterion is the D-criterion, which is the determinant of the inverse of the Fisher information matrix and minimizes the volume of the ellipsoid of concentration of the unknown vector of parameters.
One of the criticisms usually made to the Theory of Optimal Design is that a particular model has to be assumed before designing the experiment, that is before having any data. Sometimes several competing models are adequate for the same problem. In this case, a model has to be chosen after a discrimination hypotheses test. An optimality criterion to discriminate between two homoscedastic models for Normally distributed observations is the T-criterion, which maximizes the power function of the F test for lack of fit. When the rival models are nested and they differ by s parameters, another criterion for model discrimination is the Ds-one. Both T- and Ds-criteria can be applied under specific model assumptions. Differently, the recently proposed KL-criterion may be applied in a very general context: the rival models may be nested or separate; homoscedastic or heteroscedastic; Gaussian or not. The KL-criterion is based on the Kullback-Leibler divergence and it coincides with the T-criterion when the observations are normally distributed.
Dott. Antonio CANALE
Giovedì 14 Novembre 2013
Bayesian Nonparametric Models for Count Data
Count data arise in many contexts but usual parametric statistical modelling is often too restrictive and not enough flexible to capture rich multivariate interactions or complex time dependence.
Motivated by a variety of real applications including customer base management of telecommunications companies, longitudinal tumor studies, and developmental toxicity studies, this talk introduces novel Bayesian nonparametric models for count data.
Bayesian nonparametrics is a relatively young area of research which has recently received a bundant attention in the statistical literature. The considerable degree of flexibility it ensures and the development of efficient computational tools pushed both its theoretical development and its use in a number of complex real world problems.
Although Bayesian nonparametric models for continuous variables are well developed, the literature on related approaches for counts is limited.
For this reason, I will discuss recent contributions to probability mass function estimation (Canale and Dunson, 2011, JASA) and count stochastic process modeling (Canale and Dunson, 2013, Biometrika).
The main idea is to induce prior distributions on count spaces via priors on suitable latent continuous spaces and mapping functions. The procedures enjoy important theoretical properties such as large support of the prior and strong posterior consistency. Efficient Gibbs samplers are developed for posterior computation.
All the approaches are introduced and motivated by an application.
Prof. Yuzo Maruyama
Mercoledì 18 Settembre 2013
Posterior Inference and Model Selection of Bayesian Probit Regression
We study probit regression from a Bayesian perspective and give an alternative form for the posterior distribution when the prior distribution for the regression parameters is the uniform distribution. This new form allows simple Monte Carlo simulation of the posterior as opposed to MCMC simulation studied in much of the literature. We also provide alternative explicit expression for the first and second moments.
Further, under the g-priors for the regression parameters given in Maruyama and George (2011, Annals of Statistics),
we give posterior distributions and marginal densities which may be useful for model selection.
Prof. Stefano IACUS
Mercoledì 22 maggio 2013
Quanto "Big" sono i dati generati dai Social Media?
I social media come Twitter sono la fonte primaria di "big& open" data per gli statistici. Anche se i cosiddetti big data generati dai social media possono apparire ai più come rumore e intrattabili, alcune semplici idee statistiche possono permettere di estrarre informazione in modo efficiente da questo mare di opinioni.
Queste idee possono essere riassunte nei seguenti punti: i) approccio supervisionato di apprendimento degli algoritmi, ii) nessun uso di dizionari ontologici; iii) la stima diretta dell'opinione collettiva piuttosto che l'aggregazione post-classificazione di opinioni individuali.
Prof. Andrea Ongaro
Giovedì 9 maggio 2013
A new model for compositional data which generalizes the Dirichlet distribution
Compositional data consist of proportions and are therefore subject to a unit-sum constraint. Such data originate, for example, when analyzing rock compositions, household budgets, pollution or biological components and arise naturally in a great variety of disciplines.
In this seminar, a new parametric family of distributions for compositional data is proposed and investigated. Such family, called flexible Dirichlet, is obtained by normalizing a correlated basis and is a particular Dirichlet mixture. The Dirichlet distribution is included as an inner point. The flexible Dirichlet is shown to exhibit a rich dependence pattern, capable of discriminating among many of the independence concepts relevant for compositional data. At the same time it can model multi-modality. A number of stochastic representations are presented, disclosing its remarkable tractability. In particular, it is closed under marginalization, conditioning, subcomposition, amalgamation and permutation. An illustrative application to real data is shown. Finally, possible uses of the flexible Dirichlet within the Bayesian approach are touched upon.
Prof. Jordan STOYANOV, della University of Newcastle (UK),
Mercoledì 17 Aprile 2013
Some Problems from Probability, Statistics and other Branches of Mathematics
For this talk I have chosen diverse but intriguing problems from probability and statistics. Some of problems are very recent. The following topics will be discussed in detail:
• Play a lottery, you may win one million or even two millions ... Ready?
• Bernoulli LLN and Weierstrass theorem by Bernstein polynomials.
• Inference problem involving the distibutional equation X + Y = XY.
• New criterion (Hardy) for moment uniqueness of a distribution.
• Multidimensional moment problem.
• Moment determinacy of products and powers of random variables.
• Related topics including open questions.
The material will be addressed to a wide audience: from graduate students in statistical and mathematical sciences, to professionals.
Dott. Cristiano Villa della University of Kent, UK
Venerdi 12 Aprile 2013
Objective prior for the number of degrees of freedom of a t distribution
We construct an objective prior for the degrees of freedom of a t distribution.
This parameter is typically problematic to estimate, especially if the parameter space is restricted to positive integers. This is also a problem in Bayesian inference since improper priors may lead to improper posteriors, whilst proper priors may dominate the data likelihood. We find an objective criterion, based on loss functions, instead of trying to define objective probabilities directly. Truncating the prior on the degrees of freedom is necessary, as the t distribution, above a certain number of degrees of freedom, becomes the normal distribution. The defined prior is tested in simulation scenarios, including linear regression with t-distributed errors, and on real data: the daily returns of the closing Dow Jones index over a period of 98 days.
Giovedì 21 Marzo 2013
tavola rotonda sui temi del libro:
L'Italia che non cresce. Gli alibi di un Paese immobile
Carlo DELL'ARINGA, Fabio PIZZUL, Pierfrancesco MAJORINO si confrontano con l'autore Alessandro ROSINA
La tavola rotonda è moderata da Eleonora VOLTOLINA
Mercoledì 13 Marzo 2013
Olimpiadi di Statistica
Evento giunto alla terza edizione. Prove di Statistica rivolte agli studenti frequentanti le classi quarte e quinte di tutte le scuole secondarie di secondo grado del territorio nazionale
Helen MASSAM, della York University, Toronto, Canada,
Venerdì 22 Febbraio 2013
The Bayes factor and the BIC for sparse data in discrete models
The Bayes factor and the Bayesian Information Criterion (BIC) are standard tools for model selection in the class of discrete loglinear models.
For discrete Bayesian networks, using the hyper-Dirichlet as a prior, Steck and Jaakola (2002) noticed that in general, as previously observed, when the parameter $\alpha$ of the hyper Dirichlet becomes small, that is when the effect of the prior is negligible with respect to the data, the Bayes factor tends to favour sparse models. However, they also observed that occasionally and not infrequently with sparse data, the Bayes factor does not follow this behaviour but rather the behaviour of the Bayes factor varies with the data. We will analyse this situation for general hierarchical discrete loglinear models and show how the Bayes factor and BIC have to be modified for sparse data.